Banking
Today, banks are under siege from a horde of fintech companies who are more nimble than the large financial institutions. But the big banks have deeper expertise and more data, and they can win by harnessing their data and leveraging the power of artificial intelligence (AI) and machine learning to reduce costs, increase revenues, and more efficiently comply with regulations.
Syncrasy’s Data Discovery Platform provides a powerful enterprise-ready data integration, curation, data warehousing, visualization and analytics platform to underpin AI initiatives.
DataRobot’s Automated Machine Learning platform empowers existing business teams to solve analytic problems rapidly. From prospecting new corporate customers to more nimble model risk management, the banks that leverage automated machine learning will be the ones that survive the Fintech invasion.
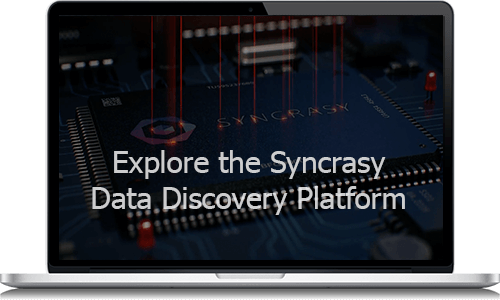
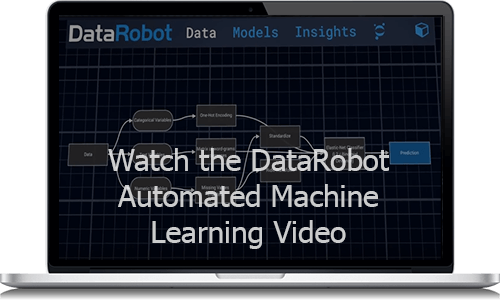
Syncrasy, DataRobot AI and Banking
As an ever increasing number of fintech companies make an already competitive market even more so, banks are being forced to look for ways to improve the effectiveness and efficiency of their business. Syncrasy and DataRobot AI helps banks improve their bottom line.
Banking Use Cases
Banks are looking to use machine learning to increase top and bottom line through gaining competitive advantages, reducing expenses, improve efficiencies and optimizing all areas of their business from risk analysis and fraud detection to marketing.
Banks are looking to use machine learning to increase top and bottom line through gaining competitive advantages, reducing expenses, improve efficiencies and optimizing all areas of their business from risk analysis and fraud detection to marketing.